Project B10 - Magnetoelectric Sensor Systems for Cardiologic Applications
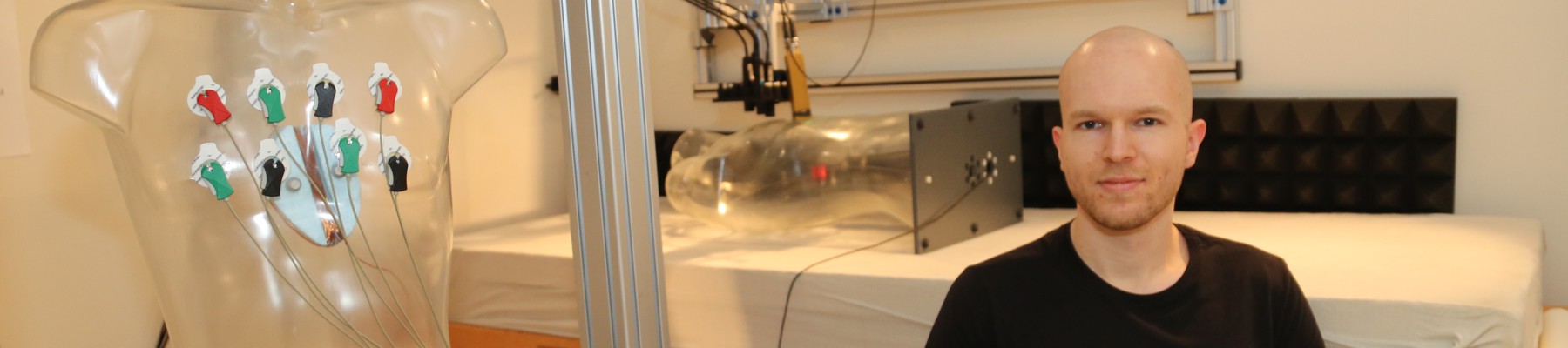
In the context of treating cardiac arrhythmias, such as those arising from tissue scarring after myocardial infarction, a precise localization of the arrhythmogenic substrate is required. To achieve this, electroanatomical mapping of the cardiac activity provides an accurate spatial and temporal reconstruction of the electrical activity within the human heart. Routinely, the mapping is performed through invasive catheter procedures. The primary objective of project B10 is to develop a non-invasive approach to electroanatomic mapping that can be employed in conjunction with invasive catheterization, with the ultimate aim of replacing it in prospective applications. The adoption of a non-invasive method offers two distinct advantages: firstly, it avoids risks associated with invasive procedures such as vascular injury, valvular injury, bleeding, or shock, and secondly, it would reduce the barriers to performing such a mapping. This perspective provides a remarkable opportunity for advancing research and facilitating disease detection and prevention. However, a significant challenge associated with non-invasive estimations lies in the fact that the number of unknown parameters of interest typically far exceeds the available measurements. If, for example, the heart has a size of about 10 cm in each direction and a spacial model resolution of 1 mm is targeted, the current density supporting points (3D), denoted by 𝑱̂(𝑖) in Fig. B10-1, would be 1.000.000 values (vectors). The number of sensors usually does not exceed a few hundred. With electrocardiography (ECG) measurements, the number of sensors (electrodes) is limited by the area of the patient’s body. Magnetocardiography (MCG) measurements do not suffer from this limitation, but even high-end setups such as at the Physikalisch Technische Bundesanstalt (PTB) are in the range of 300 sensors, Superconducting Quantum Interference Devices (SQUIDs) in this case.
Various methodologies have been employed to address this issue. Some research groups have employed highly accurate physiological models characterized by a set of parameters [Gi22]. Subsequently, to tailor the model to individual patients, an exhaustive exploration of diverse parameter combinations is performed. Ultimately, the combination yielding the optimal fit to the measured signal is selected. The advantage of this approach lies in its biological plausibility. However, the drawback is its limited scalability due to the intensive parameter search process.
Conversely, another group [Ch22] adopts machine learning techniques utilizing pairs of body surface and heart surface potentials. Through training a neural network to forecast heart surface potentials based on body surface potentials, this method accommodates numerous parameters without substantial constraints. Nonetheless, the reliance on matching training data constitutes a challenge, particularly when attempting to acquire such data for human subjects, as exemplified in their application on pigs.
In our endeavor, we are adopting a hybrid methodology consisting of time-invariant data stemming form CT or MRI-based measurements for a priori model initialization and aquisiation of magnetic and electric signals with sufficient temporal resolution on the one hand. On the other hand, data-driven model refinement strategies, as known from machine learning (cf. Fig. B10-1), are used as second part of our approach. Specifically, we are utilizing a relatively straightforward, yet robust, electrophysiological model that facilitates clear interpretability of the results. Our model is fully differentiable, eliminating the need for an exhaustive parameter search. This enables the application of gradient descent techniques for optimization. Throughout the optimization process, we exclusively rely on non-invasive measurements, thus addressing concerns related to data acquisition. In direct comparison to the aforementioned approaches, our method does not necessitate matching training data, and the patient-specific model fitting is expedited due to the utilization of gradient descent instead of parameter search. Furthermore, our state-space based model makes use of the temporal component of the measured data to improve the estimations over simple inversion approaches, which consider each time step separately. This holds the potential to enable near real-time operation, with the state estimation aspect capable of running in real time while a parallel thread focuses on model refinement. Periodically, the real-time model can be updated, providing clinicians with the most current and accurate estimation of current densities. These estimations can then be presented in a separate thread, allowing users to adjust the playback speed for more comprehensive data analysis.
Involved Researchers
Person |
Role |
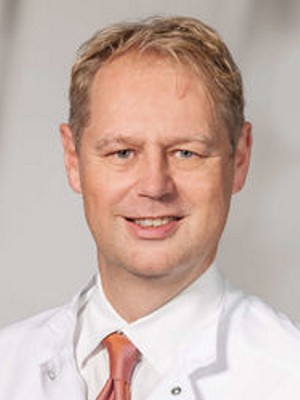 |
Prof. Dr. Norbert Frey Medicine Cardiology (Heidelberg) |
Project lead |
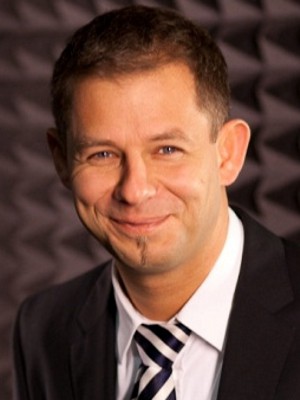 |
Prof. Dr.-Ing. Gerhard Schmidt Electrical Engineering Digital Signal Processing |
Project lead |
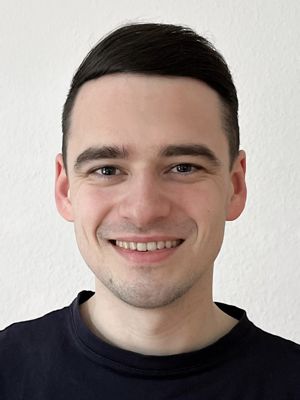 |
M.Sc. Lukas Elsner Electrical Engineering Digital Signal Processing and System Theory |
Doctoral researcher |
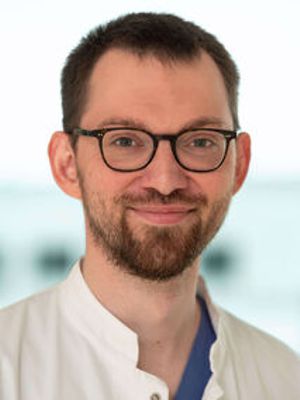 |
M.Sc. Marius Leye Medicine Cardiology (Heidelberg) |
Doctoral researcher |
Role within the Collaborative Research Centre
Project B10 will participate in the focus group F6 - Biomedical Applications and F3 - Comparison of Sensor Concepts
Concepts“.
The following table shows details about the planned cooperation of the project B10:
Collaborations |
A1 (Magnetostrictive Multilayers for Magnetoelectric
Sensors), A2 (Mechanically Soft Micro and Macrocomposite
for Wearable Devices), A4 (∆E-Effect Sensors), A7 (Electrically Modulated Magnetoelectric Sensors), A9 (Surface Acoustic Wave Magnetic Field Sensors) |
Evaluate sensors for cardiologic applications and provide feedback for sensor
development. |
A8 (Modelling of Magnetoelectric Sensors), A10 (Magnetic Noise of Magnetoelectric Sensors) |
Include sensor models and findings in forward model. |
A2 (Mechanically Soft Micro and Macrocomposite
for Wearable Devices) |
Development of flexible electroce setup. |
B1 (Sensor Noise Performance and Analogue
System Design), Z2 (Magnetoelectric Sensor Characterization) |
Work together on flexible electrodes, 32-channel MCG setup and 3D heart axis setup. |
B2 (Digital Signal Processing) and B9 (Magnetoelectric Sensors for Movement
Detection and Analysis) |
Work together in same real-time framework. Use localization algorithms for patient body
tracking. |
Z1 (MEMS Magnetoelectric Sensor Fabrication) |
Use fabricated sensor for measurement setups. |
Z2 (Magnetoelectric Sensor Characterization) |
Use sensor characterizations in forward model. |
Project-related Publications
J. Hoffmann, E. Engelhardt, M. Boueke, J. Welzel, C. Hansen, W. Maetzler, G. Schmidt: Pilot Study: Step Width Estimation with Body-Worn Magnetoelectric Sensors , Sensors, vol. 25, no. 11, 3390, 2025. |
J. Hoffmann, H. Wolframm, E. Engelhardt, M. Boueke, T. Schmidt, J. Welzel, M. Höft, W. Maetzler, G. Schmidt: A Magnetoelectric Distance Estimation System for Relative Human Motion Tracking, Sensors, vol. 25, no. 2, 495, 2025. |
J. Hoffmann, M. Boueke, E. Engelhardt, T. Schmidt, C. Hansen, J. Welzel, W. Maetzler, G. Schmidt: Proof of Principle: Full 6D Point-to-Point Motion Tracking with Magnetoelectric Sensors, IEEE Sensors, conf. proceedings, Kobe, Japan, 2024. |
E. Engelhardt, J. Hoffmann, M. Boueke, N. Frey, G. Schmidt: Enhancing Non-Invasive Electroanatomical Mapping with Dynamic Sensor Arrays, IEEE Sensors, conf. proceedings, Kobe, Japan, 2024. |
E. Engelhardt, E. Elzenheimer, J. Hoffmann, C. Meledeth, N. Frey, G. Schmidt: Non-Invasive Electroanatomical Mapping: A State-Space Approach for Myocardial Current Density Estimation, Bioengineering, 10(12), 1432, 2023. |
E. Engelhardt, E. Elzenheimer, J. Hoffman, T. Schmidt, A. Zaman, N. Frey, G. Schmidt: A Concept for Myocardial Current Density Estimation with Magnetoelectric Sensors, Current Directions in Biomedical Engineering, vol. 9, no. 1, 89-92, 2023. |
E. Elzenheimer, P. Hayes, L. Thormählen, E. Engelhardt, A. Zaman, E. Quandt, N. Frey, M. Höft, G. Schmidt: Investigation of Converse Magnetoelectric Thin Film Sensors for Magnetocardiography, IEEE Sensors Journal Print ISSN, pp. 5660-5669, 2023. |
E. Engelhardt, A. Zaman, E. Elzenheimer, N. Frey, G. Schmidt: Towards Analytically Computable Quality Classes for MCG Sensor Systems, BMT 2022, Joint Annual Conference of the Austrian, German and Swiss Societies for Biomedical Engineering, 2022. |
E. Elzenheimer, C. Bald, E. Engelhardt, J. Hoffmann, P. Hayes, J. Arbustini, A. Bahr, E. Quandt, M. Höft, G. Schmidt: Quantitative Evaluation for Magnetoelectric Sensor Systems in Biomagnetic Diagnostics, MDPI Sensors, vol. 22, no. 3, 1018, 2022. |
C. Enzingmüller, CS. Broß, D. Laumann, I. Parchmann, G. Schmidt: Magnetfelder am Herzen messen, MNU Journal, no. 2, pp. 149-153, 2021. |
J. Reermann, E. Elzenheimer, G. Schmidt: Real-Time Biomagnetic Signal Processing for Uncooled Magnetometers in Cardiology, IEEE Sensors Journal, vol. 15, issue 11, pp. 4237-4249, 2019. |